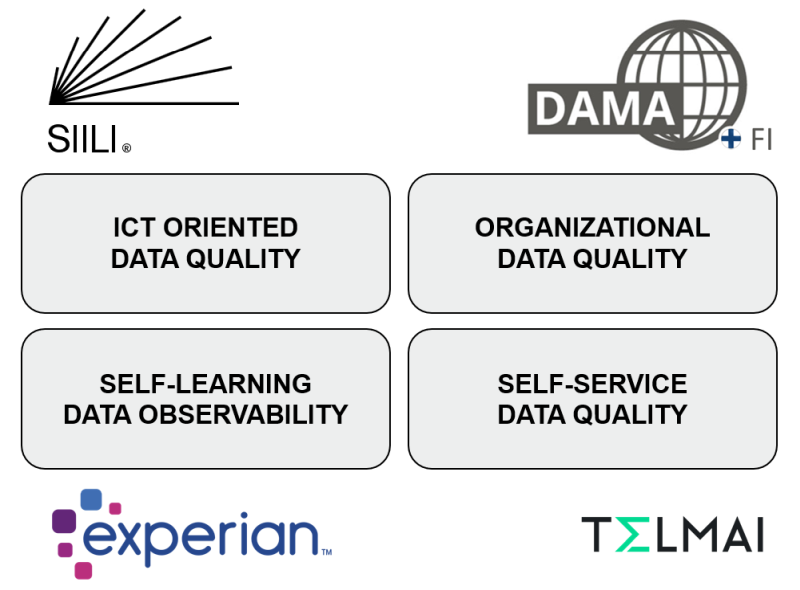
Lessons Learned from the Data Quality Management Trends and Practices Seminar
At 2nd February 2023, DAMA Finland ry organized a seminar on ‘Data Quality Management Trends and Practices’ in collaboration with Siili Solutions, Elisa, Experian and Telmai. The seminar brought together a diverse group of 40 participants, including Chief Data Officers, board professionals, data architects, and consultants. The event featured three guest presentations, complemented by an introduction and wrap-up b DAMA Finland ry.
- Trends and Practices of Data Quality Management (DAMA Finland, Sami Laine)
- Data Quality Management in Practice at Elisa (Elisa, Katri Mäkitalo)
- Self-Service Data Quality (Experian, Danny Roden)
- Self-Learning Data Observability (Telmai, Mona Rakibe)
- Wrap up (DAMA Finland, Sami Laine)
TRENDS AND PRACTICES OF DATA QUALITY MANAGEMENT
The vice-president of the DAMA Finland ry, Sami Laine, started the session by reminding about the scope and complexity of the data quality topic. Data can contain unexpected elements, omit anticipated components, convey different meanings than intended, and lose its original context throughout the extensive chain of events in business processes and system landscapes. The challenge lies in the difficulty of identifying these errors, as they may not be readily apparent within seemingly valid data. These latent errors might result from numerous vulnerabilities across various technical storage systems, software platforms, and business contexts, leading to countless potential failures.
The core part of the presentation was framed by juxtaposing Gartner’s Mode-1 and Mode-2 concepts with DataKitchen’s Front-office and Back-office concepts (Picture 1). Mode 1 is optimized for areas that are more predictable and well-understood. Mode 2 is exploratory, experimenting to solve new problems and optimized for areas of uncertainty. Back-office data teams are specialized units of technological and methodological experts. Front-offices are business users and analysts taking part in local business operations and decision-making. Each corner of the quadrant and its stakeholders has their own paradigm and the way of acting – what they aim at, how they try to achieve it and why they are very different from the others.
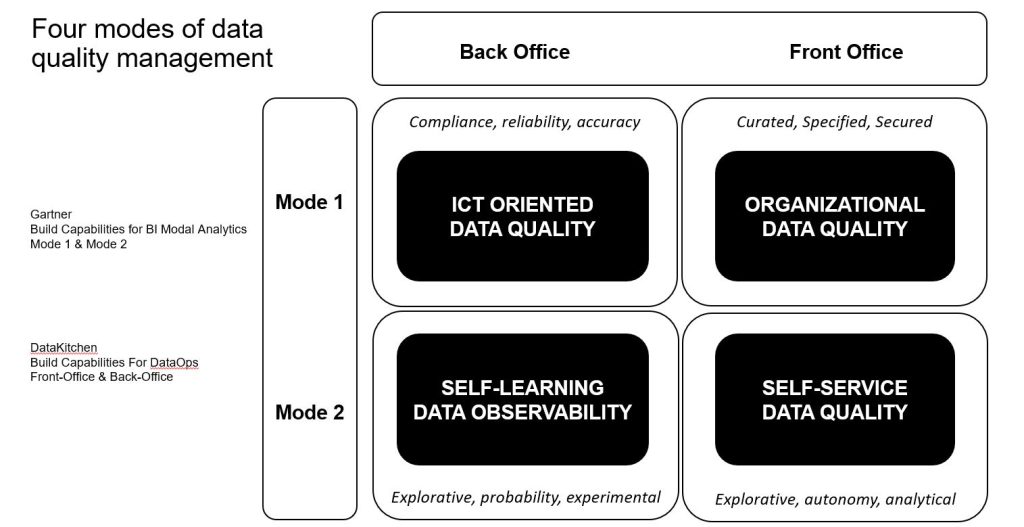
Traditionally, data engineers have been implementing data quality metrics to their databases and pipelines leading to laborious and complex shadow systems. Alternatively, business units have established data governance practices, documented guidelines and specifications, and improved communications to make the quality part of the daily business operations and long-term development projects. However, these traditional approaches have often failed to provide high quality data and they have not scaled efficiently to modern business requirements.
Due to above reasons, there is two new trends in data quality management: self-learning data observability and self-service data quality. In the first one, machine-learning systems monitor data landscape and alert if something seems to be abnormal. Data observability systems spot technically visible anomalies and trends, but they do not recognize nor fix complex pragmatic and semantics business issues that are the core interest of the front-office business users. In the latter approach, business users and analysts will be given an access to their own data to help them explore, validate and cleanse it. This approach can be agile and efficient for CRM and ERP data across the full quality cycle from prevention to fixing the issue. However, these human-insight based approaches do not scale to big data and system landscape monitoring that might be in the interest of back-office data teams. In this way, they are both needed to tackle the problems that are better handled with their strong capabilities but would most likely be missed by the other approach.
Mr. Laine ended the speech by noting that at least in Finland customer organizations and professionals do not seem to yet recognize these new trending practices. That was one of the main reasons why we chose these topics for this seminar.
DATA QUALITY MANAGEMENT IN PRACTICE AT ELISA
Elisa oyj is a Finnish telecommunication company founded in 1882. They had become aware of the data quality challenges decade or several ago. However, the initial data quality metrics and dashboards were often too technical statistics and not actionable for business users. Metrics were also laborious and slow to develop so it was difficulty to understand how wide and serious new specific data quality problems were. Business lacked visibility to actual data in their systems.
The company has been developing a wide data quality program that consists of data quality topic and tool demonstrations and freely available trainings for personnel. Data quality activities and experiments were also introduced to internal business process development meetings to bring data quality management activities closer to business and its development. In addition, data quality tools were introduced to purposes like ad hoc data exploration and error analysis, continuous quality reporting to detect misplaced PII and duplicate contact data, and to validate and fix product data issues such as categorization errors. However, it’s not yet clear what kind of organizational structures, technical tools and practices work best for each case since the thing that works somewhere does not always work everywhere.
The main lessons were that the data quality should be built into the business processes and systems. The errors should be prevented and fixed at the source and data quality should be collective organizational intelligence – collaboration, communication, conceptualization and continuous learning.
SELF-SERVICE DATA QUALITY WITH APERTURE DATA STUDIO
Experian is one of the oldest and biggest data service companies in the world and data quality has been their core business issue for hundreds of years. Their subsidiary called Experian Data Quality has been providing data quality services and tools for decades. Particularly, they have been focusing on data quality management task acceleration and business-user friendliness. The Aperture Data Studio data quality platform provides business-users and analysts data accessibility, exploration, and preparation capabilities with a user-friendly low-code interface.
The presentation walked through four phases of typical data quality management scenario: 1) investigate data landscape, 2) assess specific issues with automatable metrics, 3) improve data quality with advanced functionalities, and 4) control data quality by setting up data quality firewalls and continuous monitoring dashboards. For example, the tool helped end users to connect to a wide variety of data sources, profile their contents and tag the source data with labels such as PII. During assessment, the Aperture can suggest quality metrics based on profiling results but let’s users to add also custom metrics. To actually improve data quality, the tool has advanced data preparation, cleansing and enrichment functions that help end users to change data to their desired state.
In the above way, the demonstration illustrated how the Aperture Data Studio can accelerate data quality management in each step. These features were highly visual and easily accessible by business users as well as powered by machine-learning and automation features.
You can learn more about the Aperture Data Studio by joining the webinar to see it in action here: (Link to the forthcoming Aperture Data Studio Demonstration).
SELF-LEARNING DATA OBSERVABILITY WITH TELMAI
Telmai is a new company that has built the Telmai data observability platform for the Data Teams to proactively detect and investigate anomalies in real-time. The challenge has been that modern data landscapes are crowded with hundreds of software systems, terabytes of data and constantly changing technological landscape have created a scalability problem for traditional data quality tools.
In the beginning, the founder and CEO of Telmai, Mona Rakibe, explained the differences between traditional data quality and more recent data observability approaches. The main message was the modern data observability tools leverage ML and statistical analysis to learn about data and predict future thresholds and data drifts. Their aim to reduce the need for human effort and provide automation for large-scale data and metadata monitoring. At the same time, the scope of the data observability genre varies quite a lot. Some tools focus more strictly on data and metadata monitoring while others might have capabilities to monitor data usage and cost optimization.
The demonstration illustrated how the system connects to sources, monitors the data sources and metadata to learn more about them, and then presents visual statistics and trends about its findings. The core parts of the system were its ability to send alerts about its findings and to help data team analyze what happened and when across the system landscape. One of the most interesting parts of the presentation was to see how the system was tuned by configuring the training data to optimize it. After all, data observability systems such as these must be tuned to avoid false positives and false negatives and keep the true positive alerts at optimal level to save time and effort for valid issues.
You can learn more about the Telmai data observability platform by requesting a demo here: (Link to Telmai Data Observability Demo).
SUMMARY
The traditional data quality management – based on technical database content metrics and dashboards, developed manually with scripts and ETL tools by ICT teams – just does not scale to the modern technological environments and business needs. There’s just never enough time or resources to build metrics for all the potential issues. This was the core message of all presentations although they ended up promoting a different approach and lessons learned.
First, Elisa telecommunication company noted that they had gained benefits from self-service data quality management in comparison to traditional ICT lead efforts. However, they emphasized that data quality should be built into the business processes and systems in the first place and at the source. In addition, there does not seem to be a silver bullet for perfecting data quality – approaches, practices and tools that work somewhere do not work everywhere and for all data quality problems.
Second, Experian demonstrated how a modern self-service tool like Aperture Data Studio can accelerate data quality management with built-in automation and non-technical transparency in each step of the quality management cycle. In this way, self-service can empower business to take control of their own data and processes while saving in ICT costs and speeding up the root cause analysis and impact estimation processes. The benefits of full-scale data quality tools are that they cover the entire quality cycle: define, measure, analyse, and improve. Data preparation and exploration capabilities are needed to recognize, fix, and prevent more complex data quality issues that are best handled by senior business users who know how the business and its works.
Third, Telmai emphasized that human effort just does not scale to the needs of modern data-driven business. Rather than trying to manually explore data sets and implement quality metrics by own hands data teams should look for data observability tools that leverage ML and statistical analysis to learn about data and to predict future thresholds and data drifts. The benefits of state-of-art data observability tools are that they reduce the human effort in designing, implementing, and maintaining data quality rules and metrics. These systems focus only to measurement and analyse steps but do it with a much wider support for different data types and larger volumes than traditional data quality tools. These modern data observability tools are needed by technical data teams so they can autogenerate quality monitoring across rapidly changing data and system landscapes.
Sami Laine, Vice-President, DAMA Finland ry